SIRIUS
LEP (Limited English Proficiency) Healthcare Assistant
ROLE: DESIGNER, RESEARCHER
08/20 - 12/20
Academic project completed at Cornell University with Xirui He, Danni Ji, Yuchang Zhou
Instructor: Professor Cristobal Cheyre Forestier
ABOUT THE PROJECT
Improving the accessibility of medical resources for under-served communities with technology.
With “Making the World More Just and Equitable” as our high-level target, our team chose to tackle the problem of healthcare disparity with a creative technology solution to improve the accessibility of medical resources for the under-served population.
01 FINDINGS
Why is this Problem Important?
Our problem space focuses on under-served communities not getting equal healthcare accessibility as advantaged populations. We hope to design a solution that could effectively improve the accessibility of healthcare services for less advantaged groups with technology that could be applied in realistic settings, which would essentially assist the under-served community to receive better treatment.
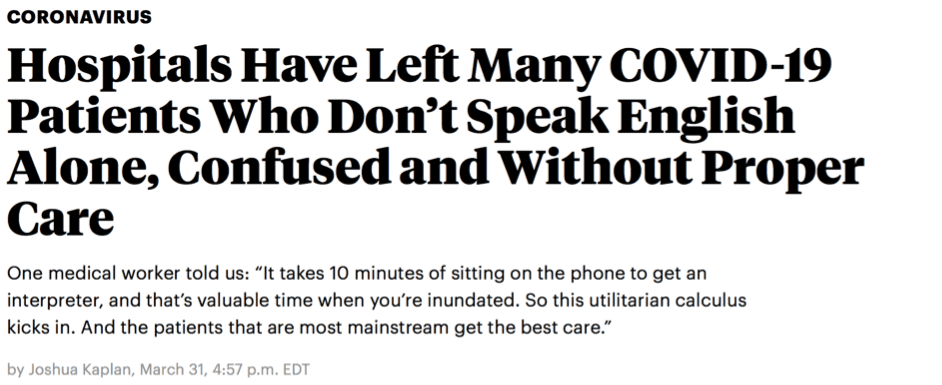
Narrowing Down the Problem Scope
Not all differences in health status between groups are considered disparities, but rather only differences that systematically and negatively impact less advantaged groups are considered disparities. Much of the current research focuses on unequal health treatment because of racial and ethnic disparities in the community.
Other important factors to health disparities we found include food insecurity, limited access to transportation, housing instability, lack of insurance coverage, and cultural, linguistic barriers. When caring for the under-served community, an important theme is the inadequate patient resources and provider resources. It might be difficult for patients to access educational materials, especially non-English ones. Many local organizations target specific racial groups or issues to help the under-served communities get more information and better healthcare. However, a large portion of these materials is information-based and is inefficient to use.
In addition to literature research, we conducted four interviews with researchers and experts from Cornell Center of Health Equity.
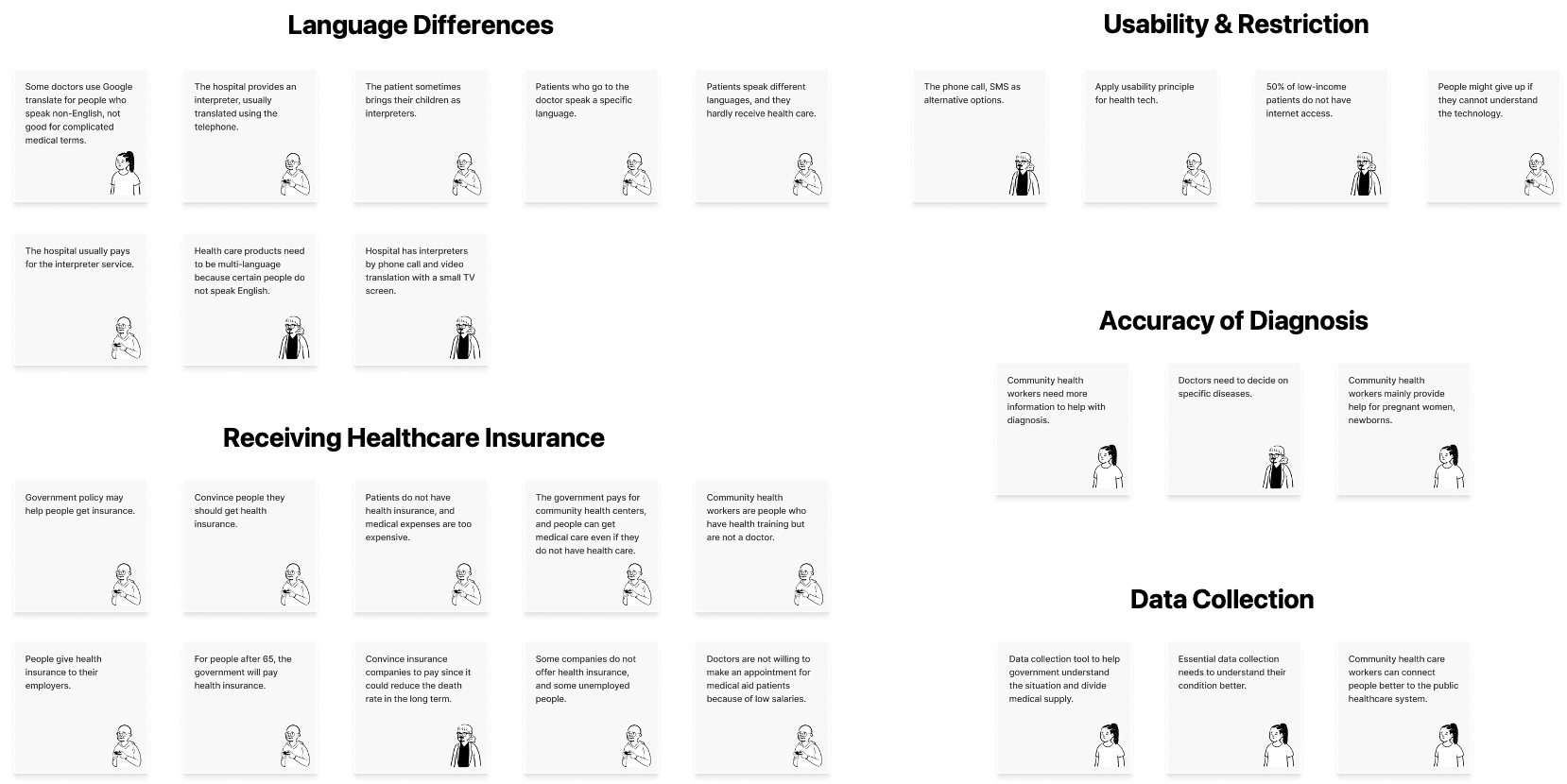
After the initial research, we narrowed down the topic to improve the hospital service for patients with limited English proficiency. After we narrowed down our problem statement and target population, we conducted more literature review to gain a better understanding of the existing solutions and technology used.
Interpreter
Higher accuracy, but usually a long waiting time, and there are not enough interpreters, especially for uncommon languages.
Crowdsourcing
By soliciting multiple translations and redundantly editing them than selecting the best, it is possible to get high-quality translations without professional medical translation.
Machine Translation
Although the direct narration of the content can be exact, there are still limitations in the expressions of meaning.
Goals
Reduce the waiting time caused by insufficient professional interpreters.
Decrease medical error rate related to language limitations.
Provide multi-language technology service while having alternative options, including text messages and phone calls for patients with limited internet access.
Protect privacy and build trust for patients and healthcare providers.
02 DESIGN
Initial Exploration & Feedback
We hope to solve the shortage of translators by recruiting volunteers and through appointment arrangements to increase translation staff's work efficiency. At the same time, we arrange a professional translator and a volunteer in each translation work to supervise the translation results and quality of each other to ensure the accuracy of the translation.
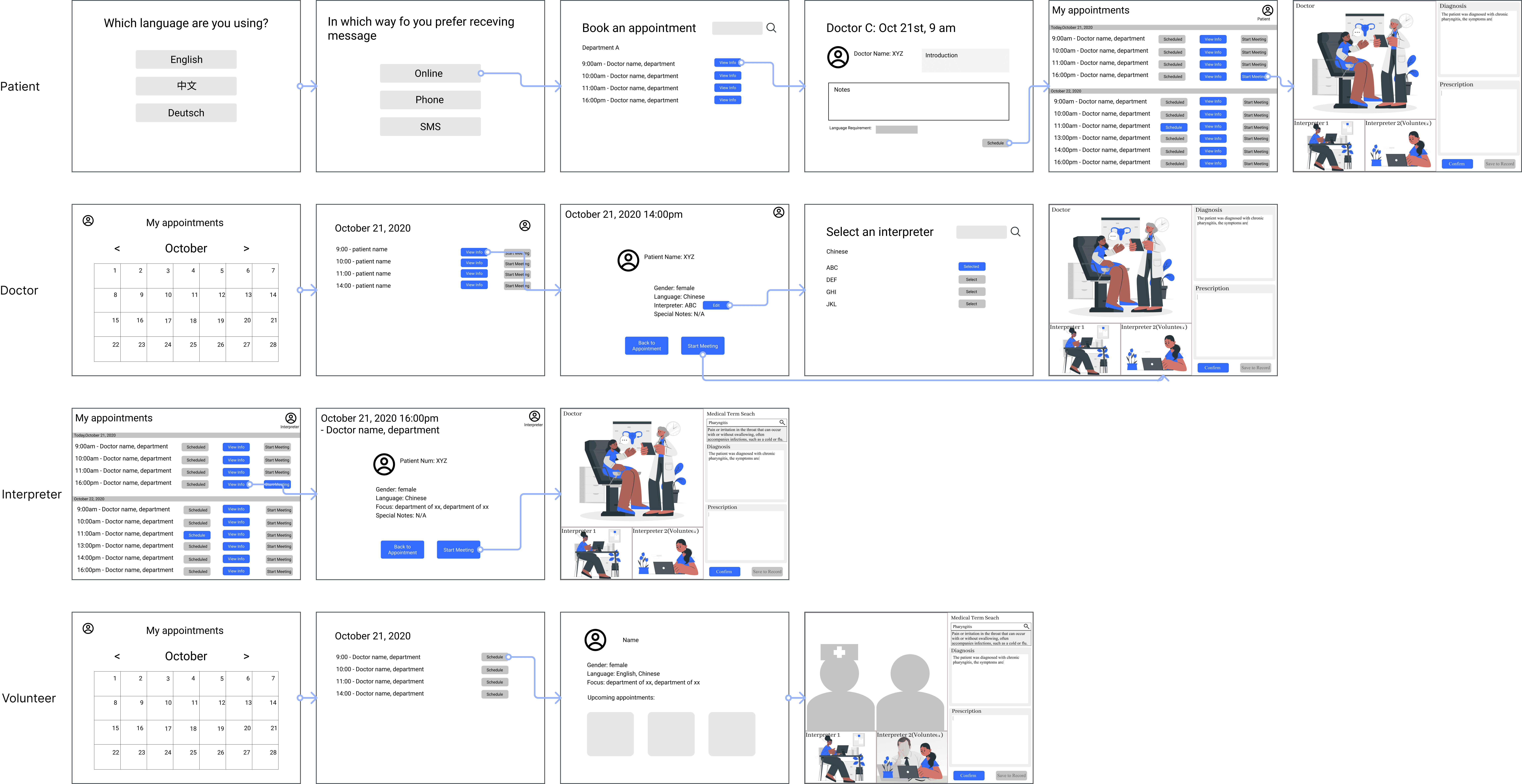
Primary Functions
Patients can make appointments online, by telephone, or by SMS.
Doctors can view the content of the appointment and request the participation of translators and volunteers. Translators and volunteers can agree to join the meeting.
A search function to facilitate interpreters and volunteers to confirm some professional medical terms further.
A function for volunteers to record relevant diagnostic results and prescriptions. This information will be sent to the patient after the meeting.
Feedback from Professional Healthcare Workers
“You will not be able to keep the number of volunteers to participate in the meetings. In addition, having both a professional interpreter and a volunteer join in a meeting does not solve the problem of insufficient translators to a large extent but slows down the interaction.”
Logic Behind Design
Combined Crowdsourcing with AI Translation
By including these two resources in our product, we mainly solve the problem of not having enough interpreters because it is possible to obtain high-quality translations from non-professional translators. The cost is an order of magnitude cheaper than professional translation.
Translation Ability Test
The crowdsourcing staff needs to pass this test to serve as a real case. This test will give users some random medical-related sentences and ask them to finish translation in a short time, and then AI will check their accuracy level.
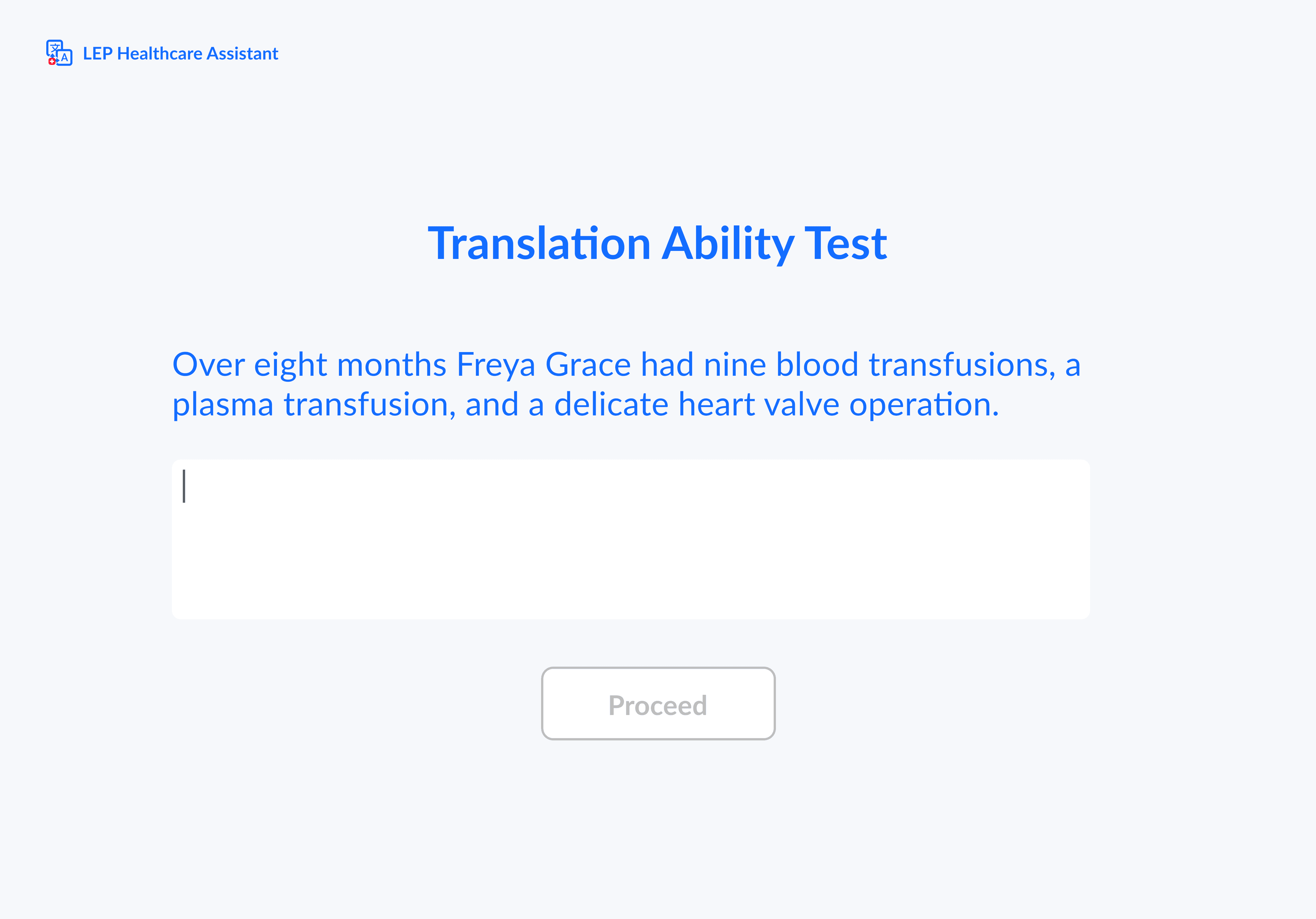
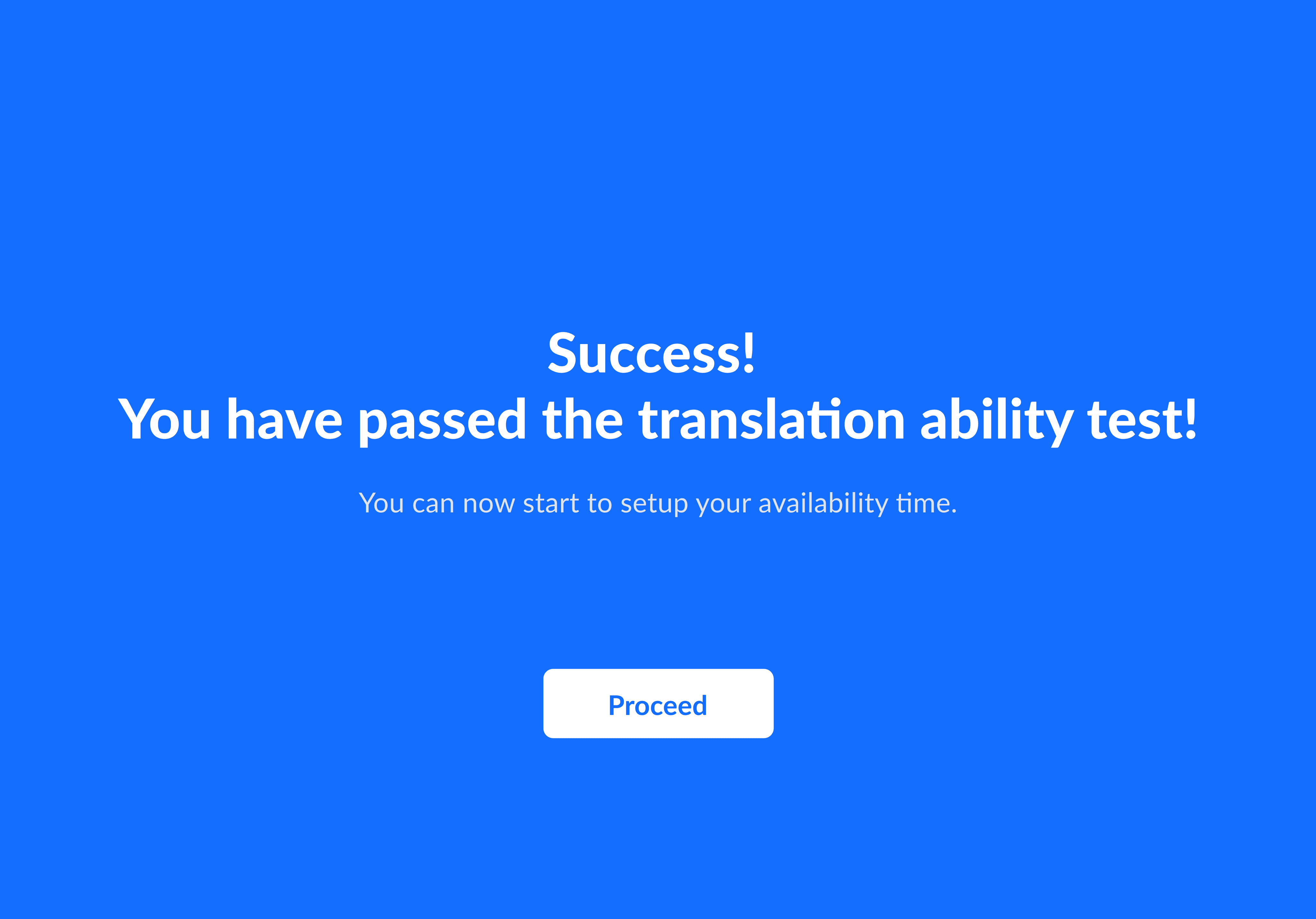
Expect Five Crowdsourcing Staff to Work Together when Doing the Text Translation and Have AI Automatically Check the Translation Quality
We minimized the possibility that individuals caused translation errors.
Privacy Protection
In the initial video process, patients can only choose to turn their videos on or off, but this will also affect the accuracy of the translation at certain times. We took this into consideration and added a design that allows patients to mosaic their faces. This not only protects the privacy of patients but also allows translators to receive their physical expressions better.

Increased Contact Efficiency with Professional Translators
While connecting with the interpreters, the system will quickly calculate based on the interpreter's work schedule, availability status, and if the interpreter fits the patients' language needs. Based on the calculation result, there will be a list of interpreters the system is trying to connect with. For each interpreter, we will wait for 25 seconds for the interpreter to accept the translation request.
Finalized User Flow
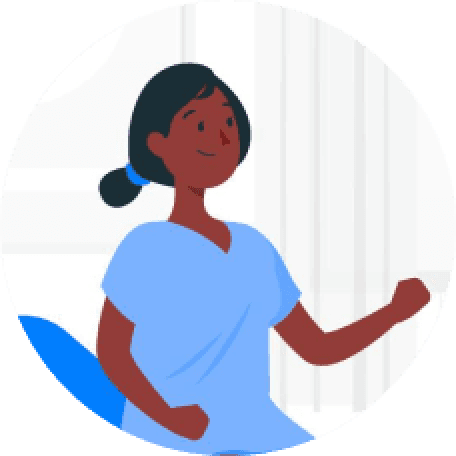
Patient
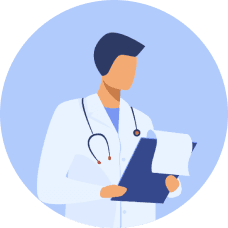
Doctor
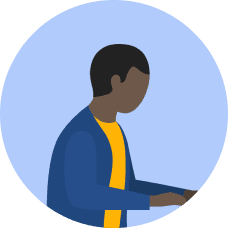
Crowdsourcing
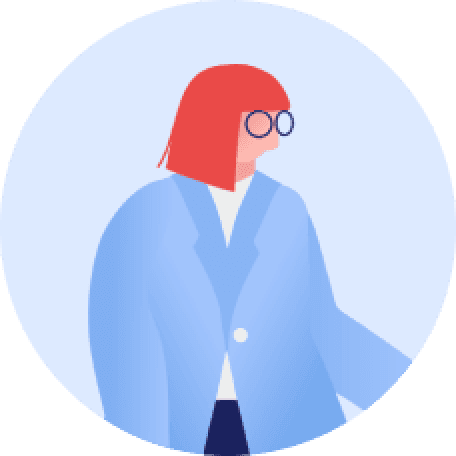
Interpreter
Change language
View appointments
Take translation ability test (first time only)
Setup Work Schedule
Input basic informations
Choose crowding or interpreter
Setup work schedule
Book appointment
M
E
E
T
I
N
G
Review record and confirm translation results
Review record and confirm translation results
See appointment records
Change from crowdsourcing to interpreter
Submit Record
04 PROTOTYPE
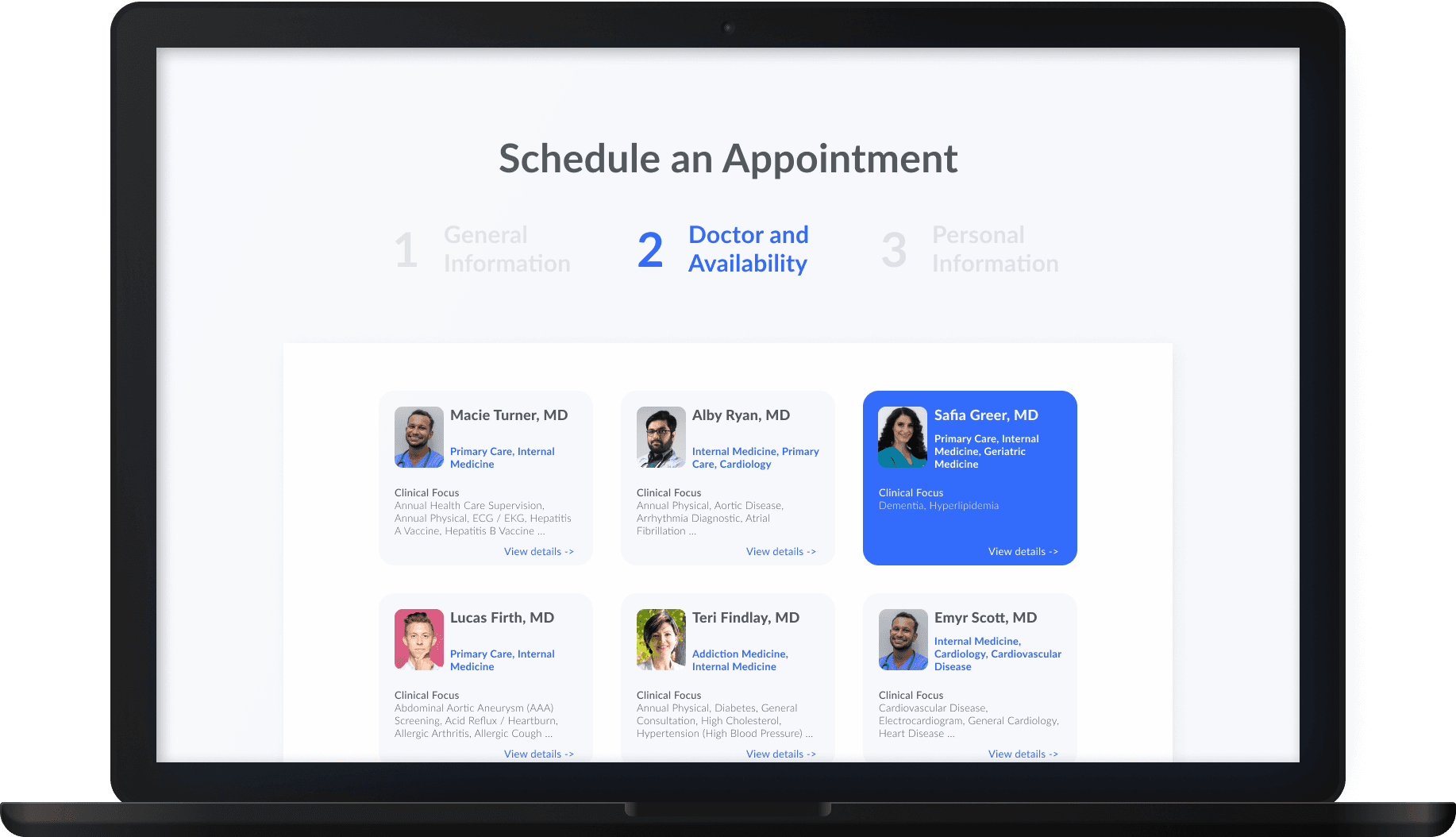
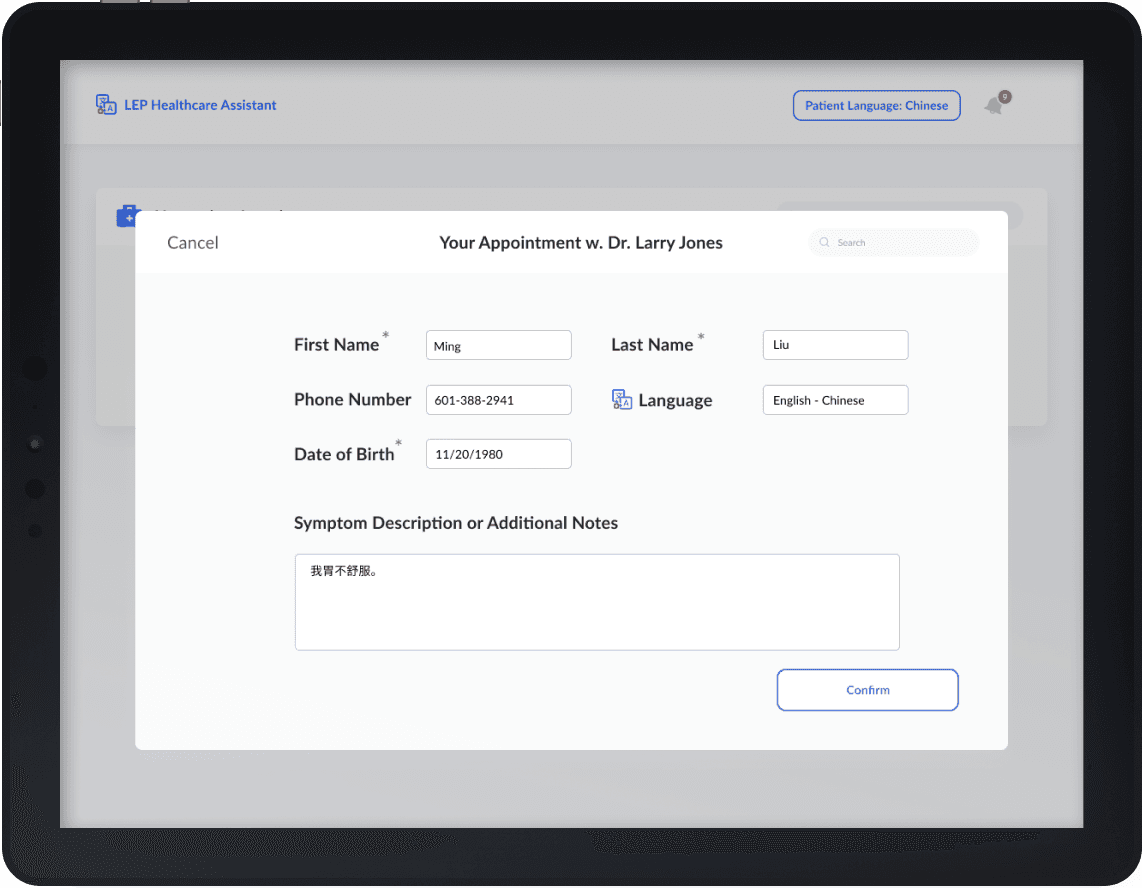
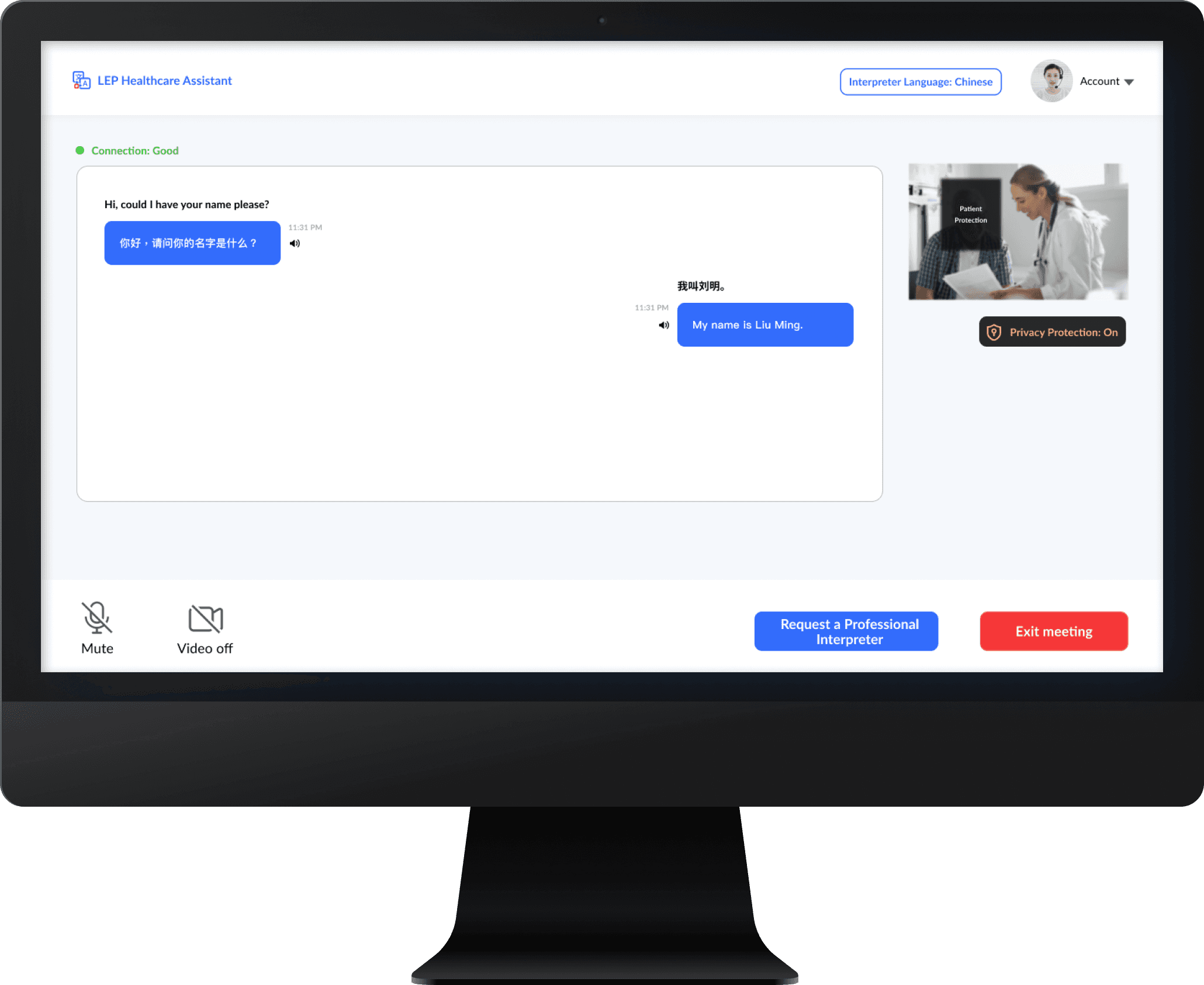
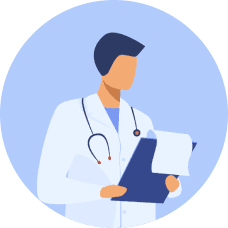
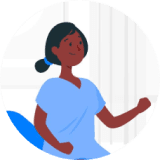
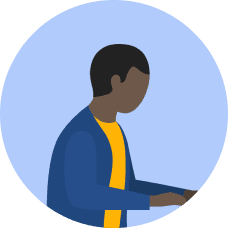
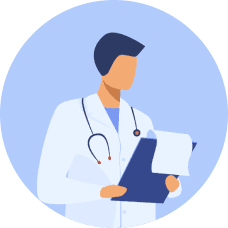
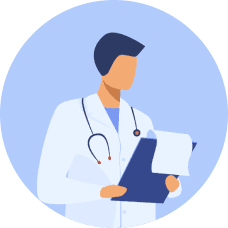
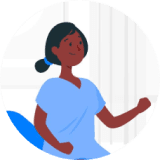
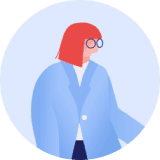
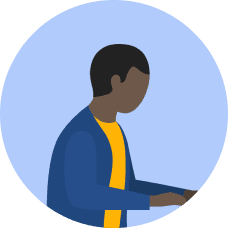
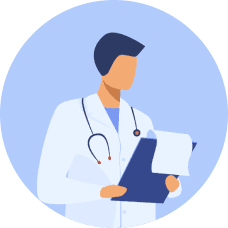
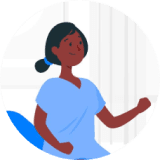
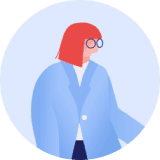
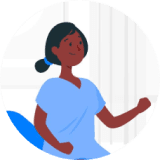
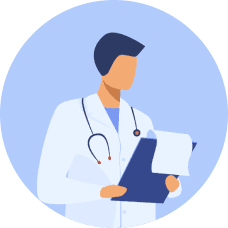
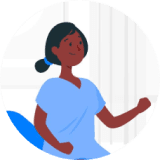
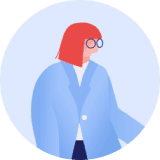
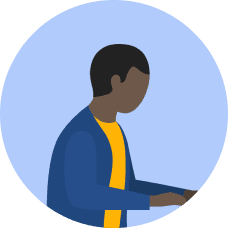
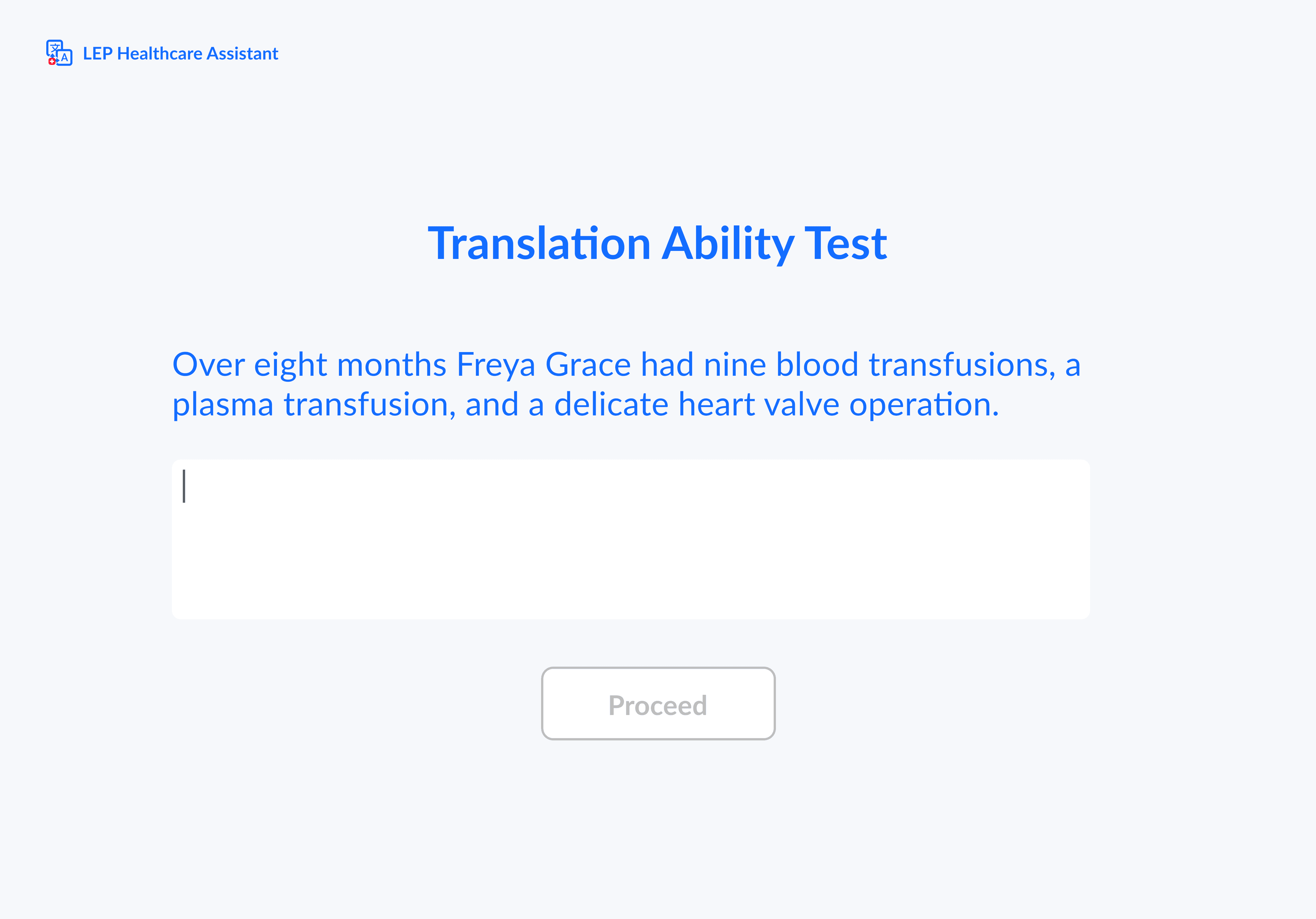
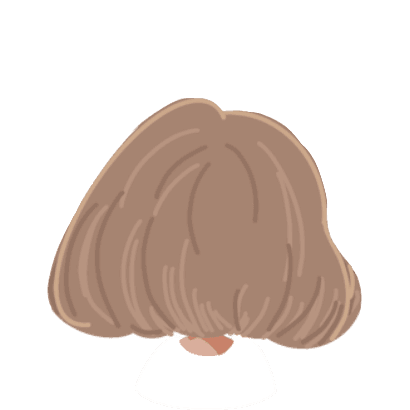
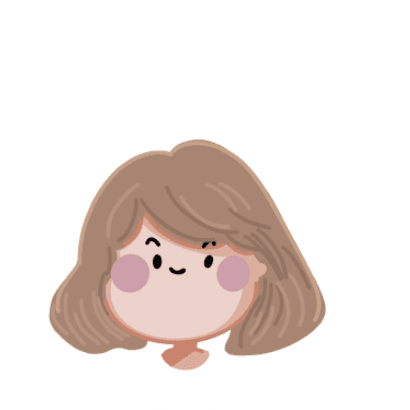
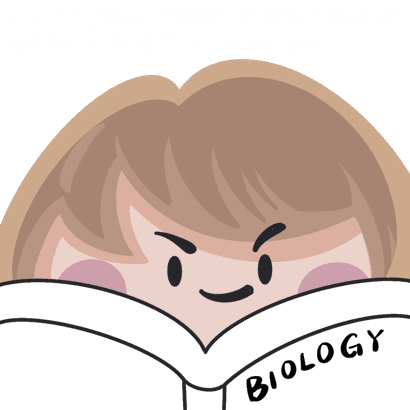
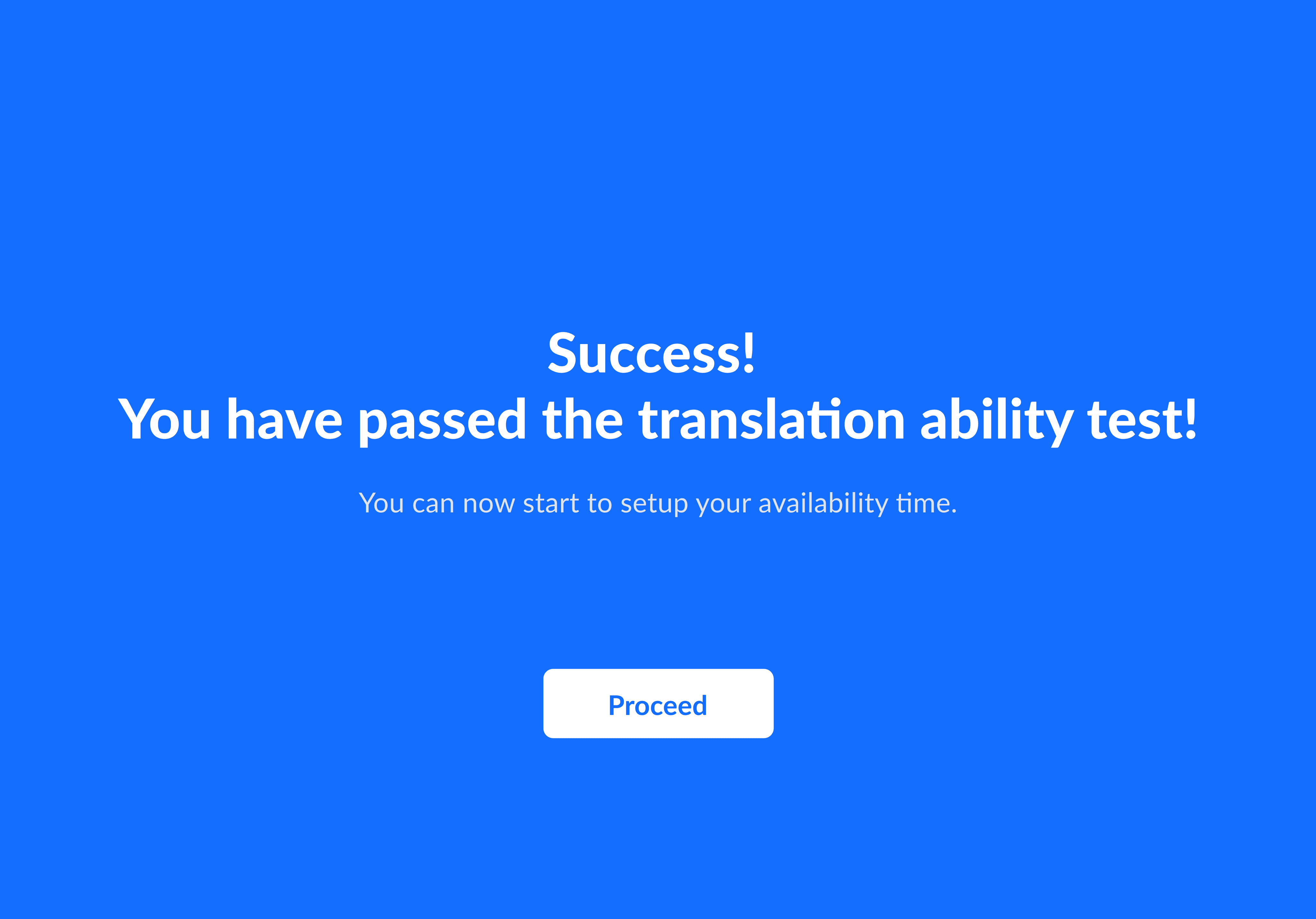
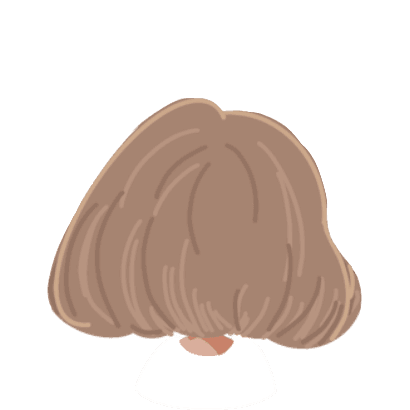
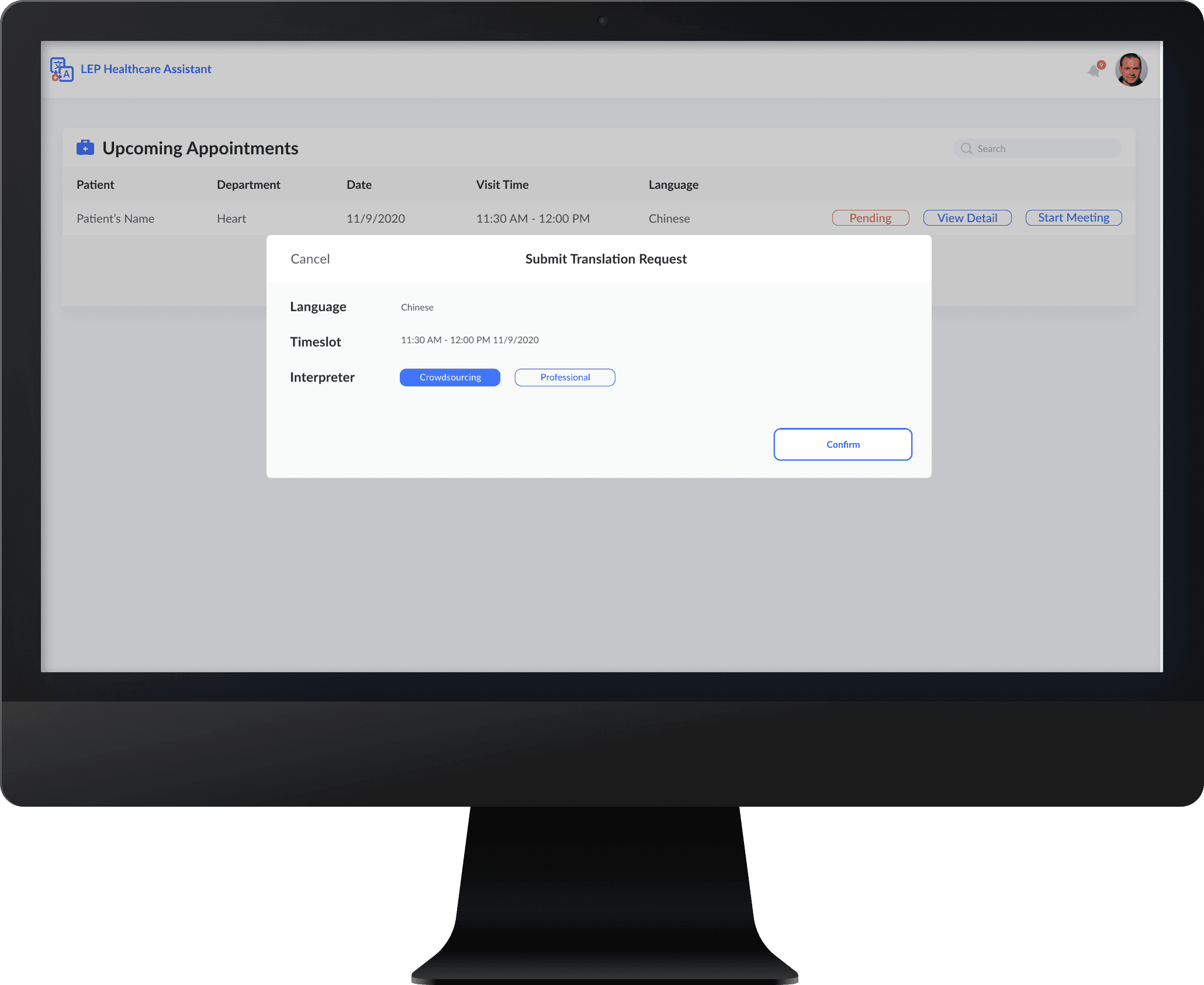

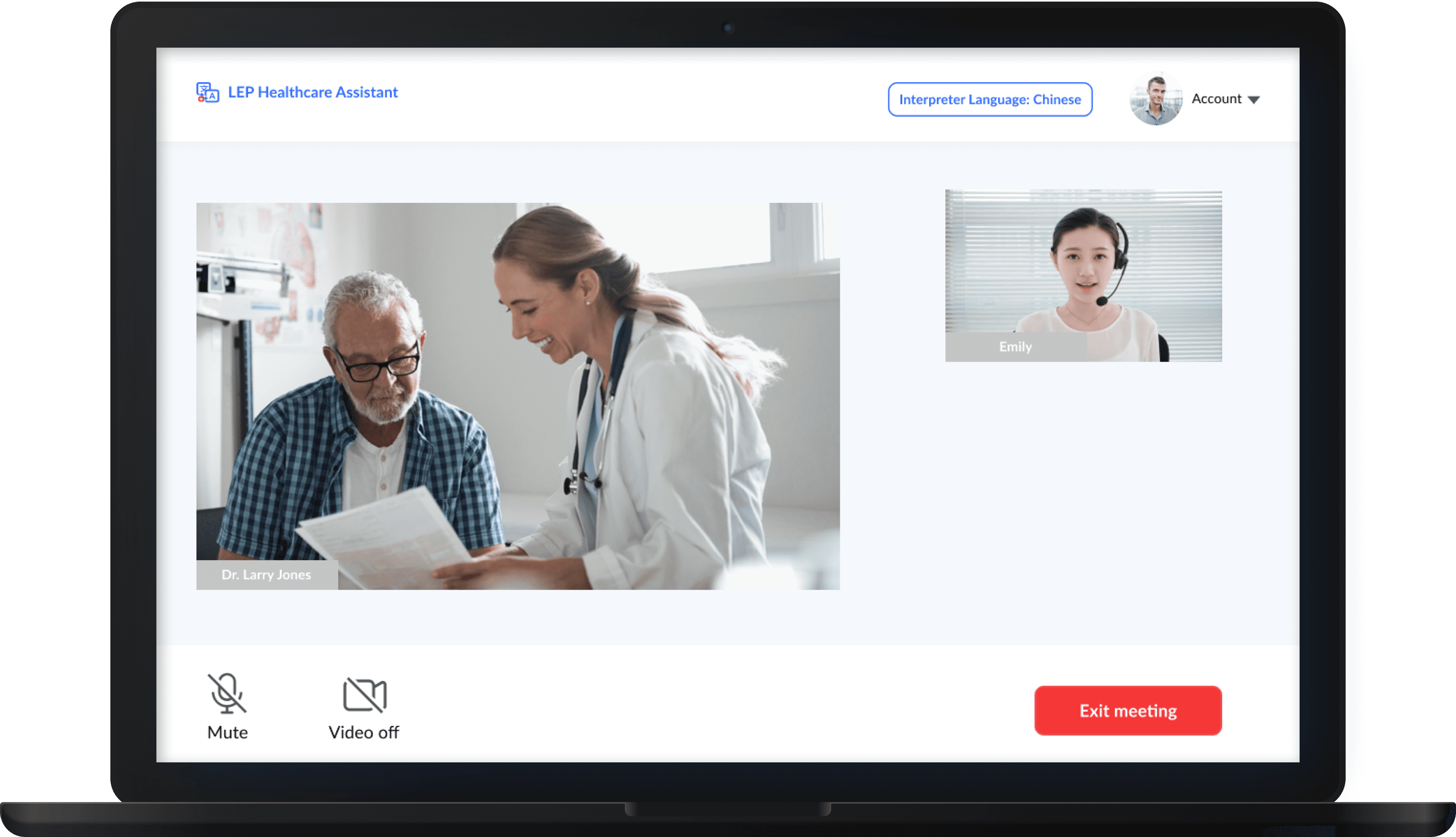
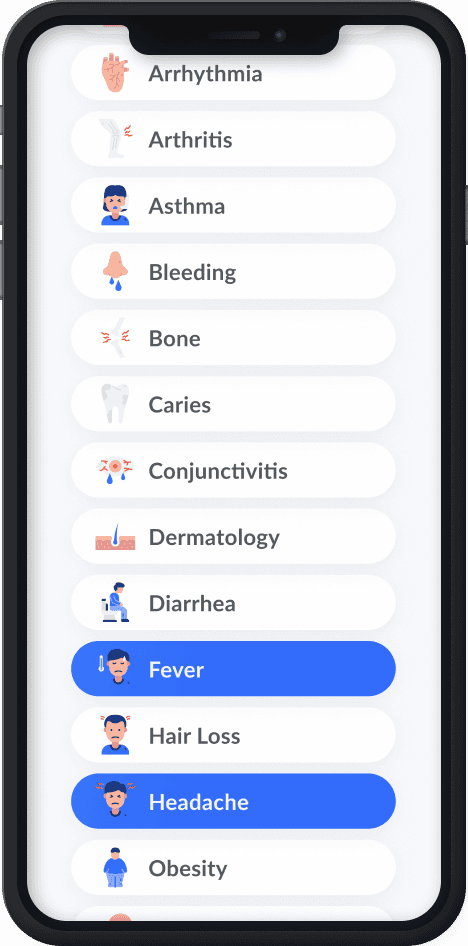
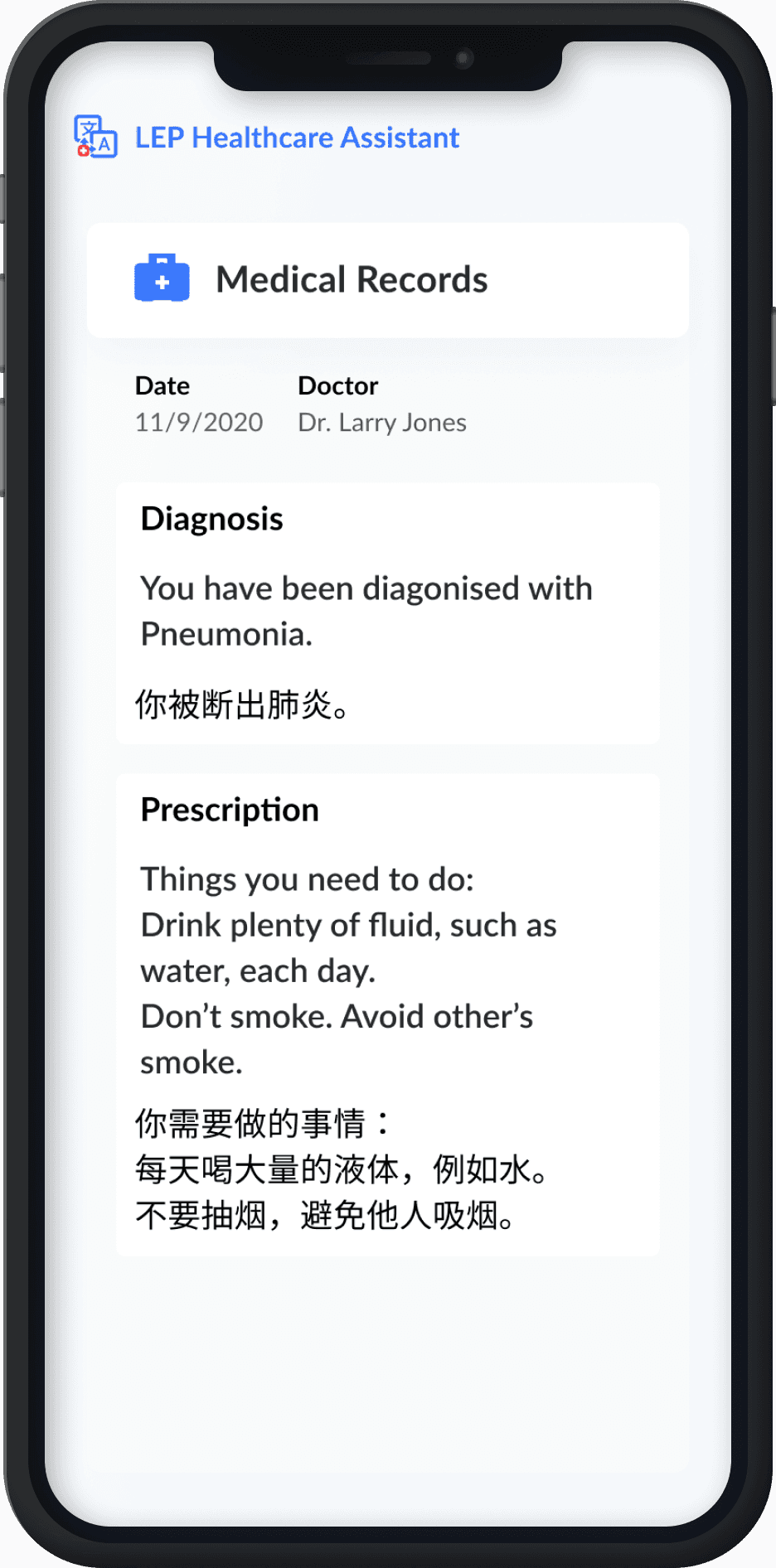
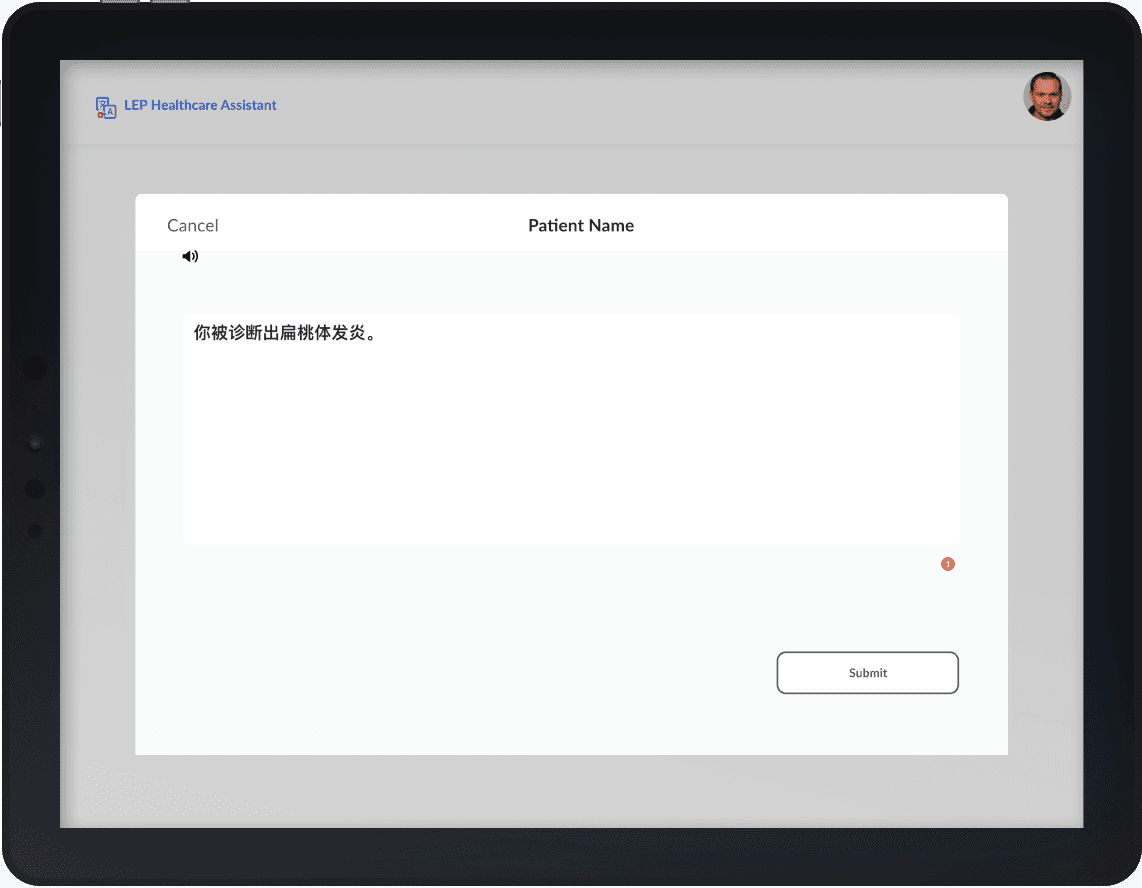
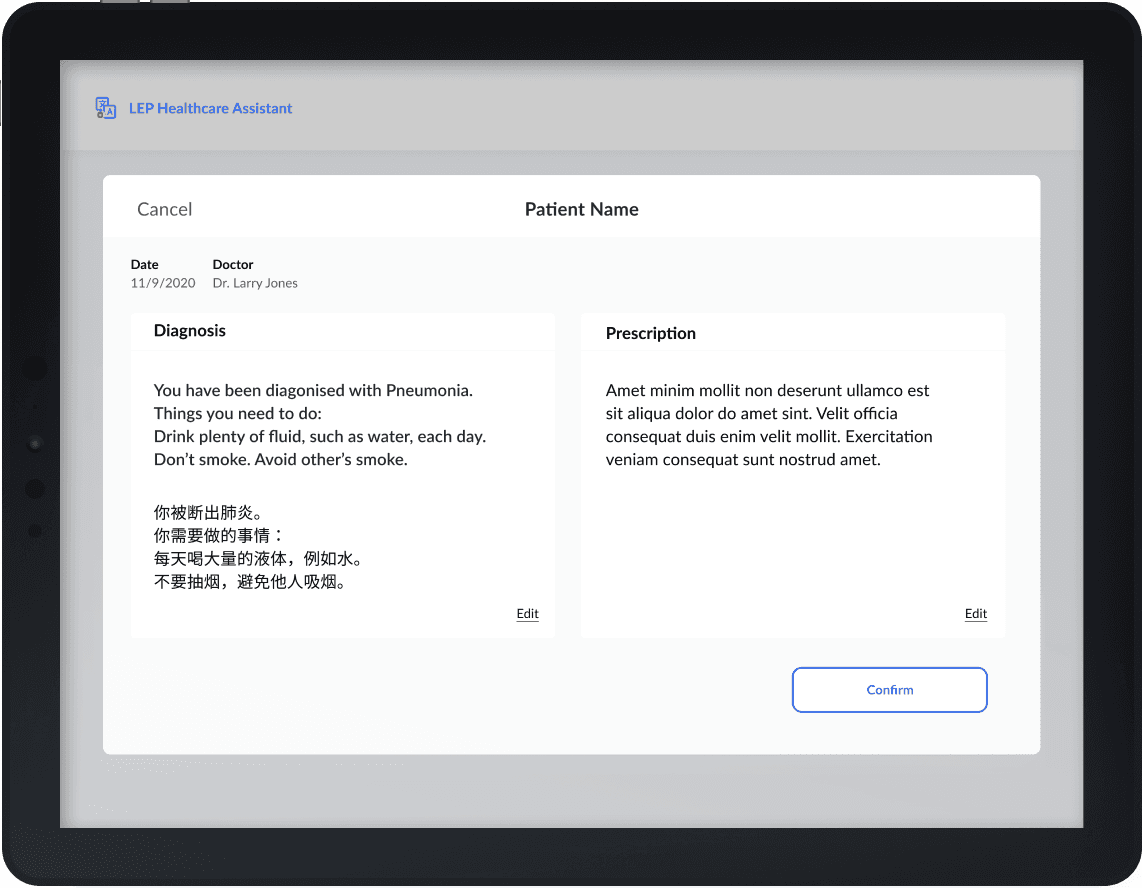
04 EVALUATION
Wizard of Oz
In addition to the user, three people will be participating in the interview process: the doctor, the interpreter, and the crowdsourcing staff, and the other will be a notetaker. The person acting as the doctor will face the user, who will pretend to be a patient, while the remaining two will participate remotely.
We recruited two participants who are native Chinese speakers and do not speak or understand English well, one participant in the medical industry, and one participant who had experience translating for medical appointments.
Task 1: Rash
This is a milder symptom, crowdsourcing staff will translate for him throughout the process.
Task 2: Abdominal Pain
The doctor underestimated the severity so crowdsourcing staff will be switched to professional interpreters during the diagnosis process
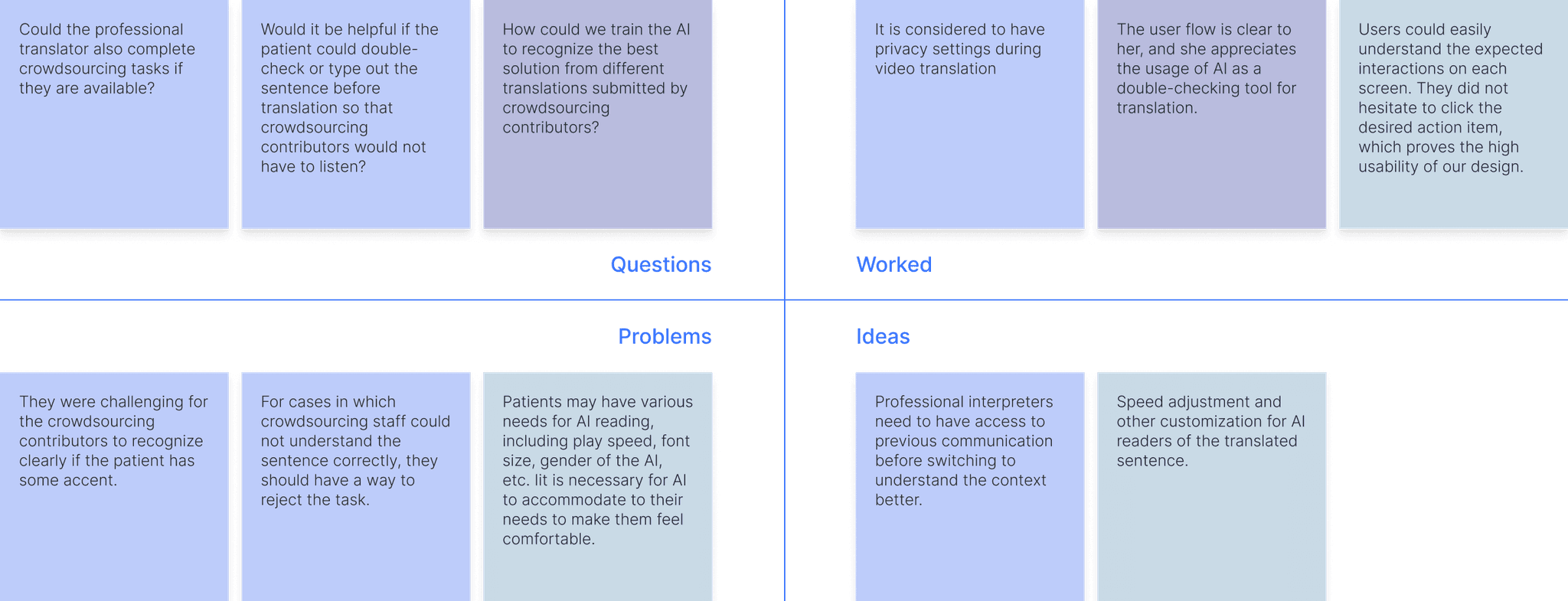
Insight
The interpreter needs to understand the context to translate accurately quickly.
LEP patients need more assurance about the accuracy of translating content.
Crowdsourcing staff should have a way to reject the task.Patients have various needs for AI reading, including play speed, font size, gender of the AI, etc.
Improvements After Evaluation
Professional Interpreters need to Know the Context and Discussion before Switching.
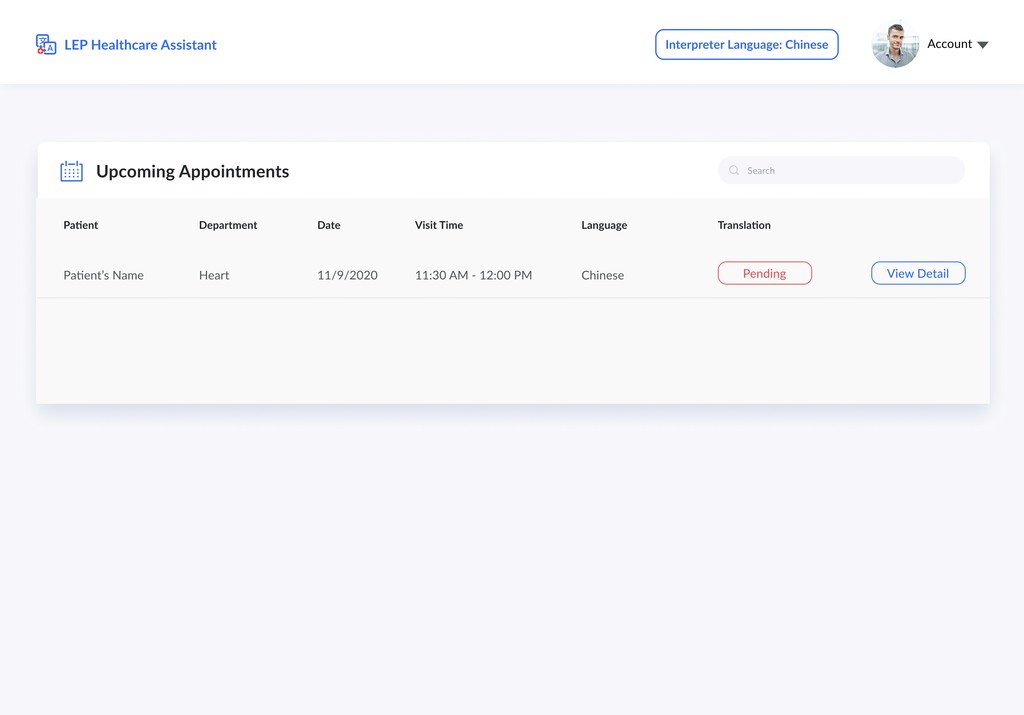
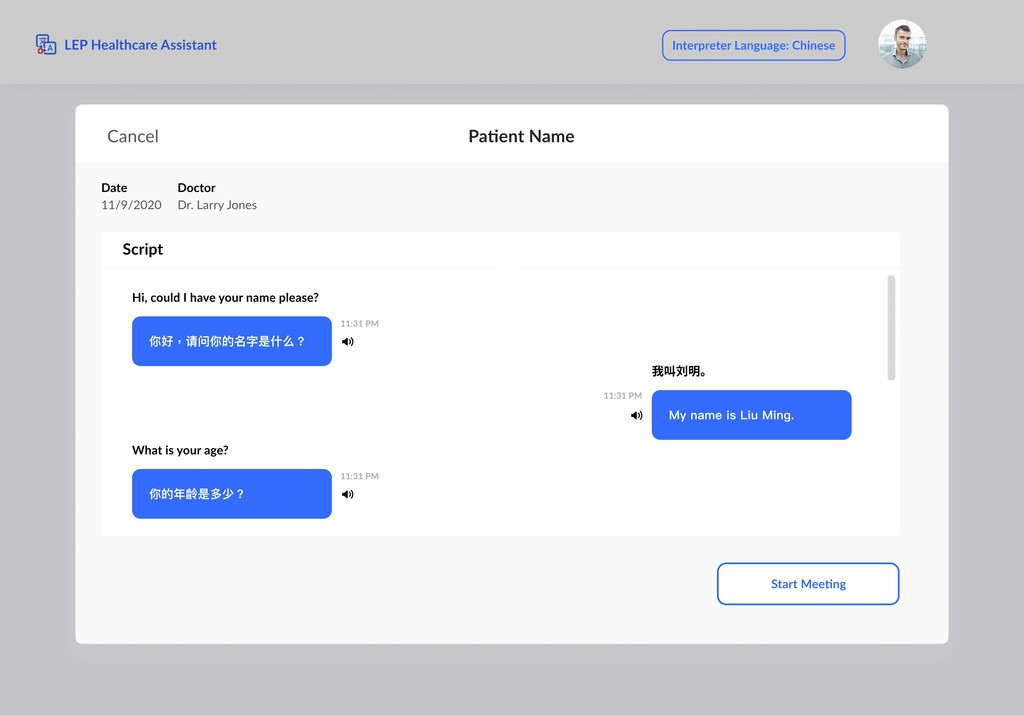
Patients need Customization to Understand AI Reading.
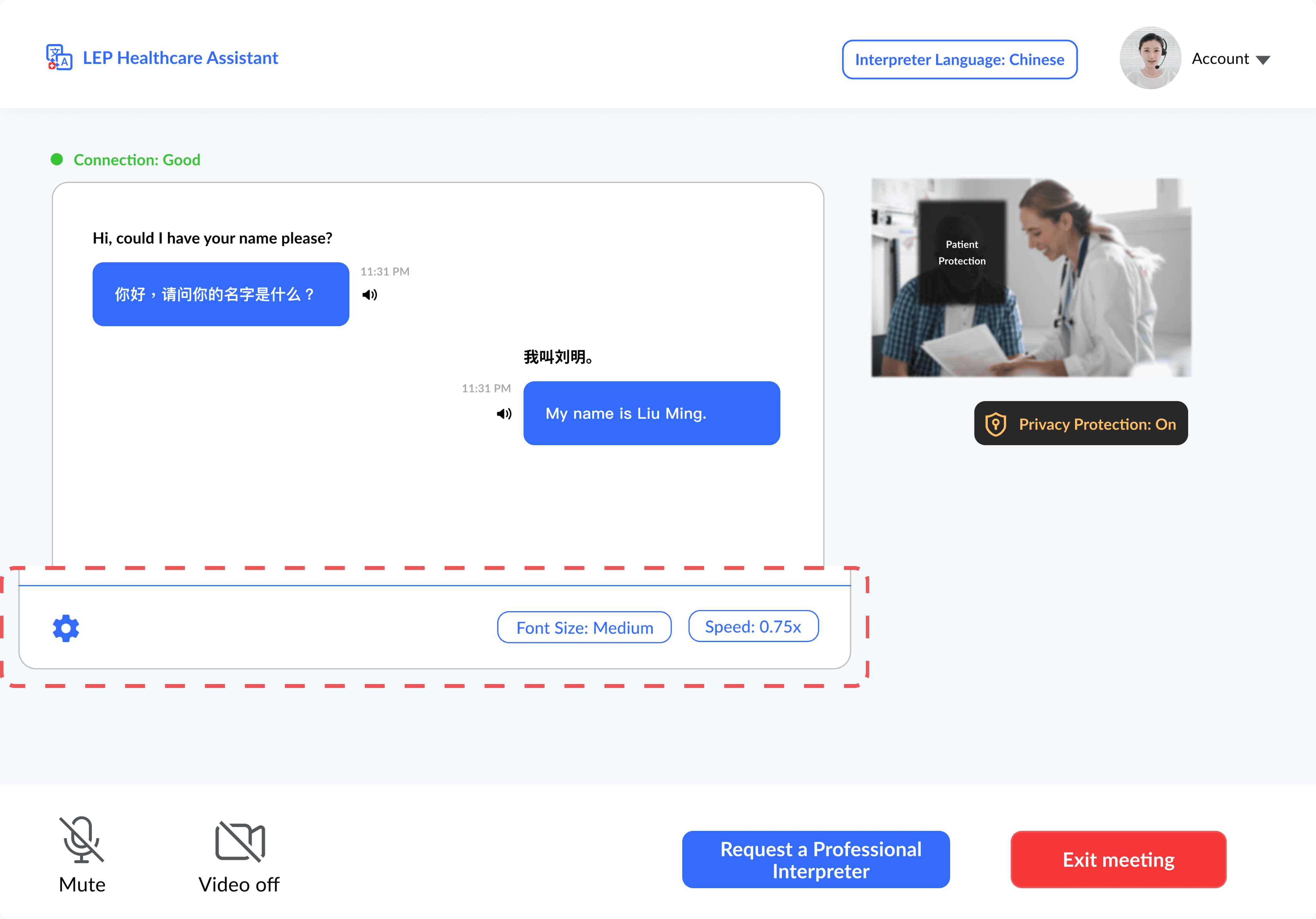
How to Train the AI?
We expect the AI to train by a series of translation scripts for medical cases and some databases/dictionaries containing medical terms that are frequently used.
How to Solve Problems during Crowdsourcing?
If the crowdsourcing contributor could not recognize the sentence or do not know the correct translation:
They should have the option to reject the task.
If the five submitted translations for one sentence are significantly different from one another and AI could not decide on the optimal solution:
Our platform would hire a certain number of experts (professional interpreters) as the final decision-makers. They would receive unsolved tasks from the system under the circumstance described above and give the final solution.
If the professional translator would like to work on crowdsourcing tasks in the available time:
They could turn on their availability for crowdsourcing, and they would be able to receive tasks.
How to Solve Problem During Crowdsourcing?
If the crowdsourcing contributor could not recognize the sentence or do not know the correct translation:
They should have the option to reject the task.
If the five submitted translations for one sentence are significantly different from one another and AI could not decide on the optimal solution:
Our platform would hire a certain number of experts (professional interpreters) as the final decision-makers. They would receive unsolved tasks from the system under the circumstance described above and give the final solution.
If the professional translator would like to work on crowdsourcing tasks in the available time:
They could turn on their availability for crowdsourcing, and they would be able to receive tasks.
How to Promote Our Product?
Cooperate with the hospital by putting our product information on their website. One feature can be implemented is that while we found that the users' default language setting is not English, there will be a pop-up screen asking users if they need our service in their appointment.
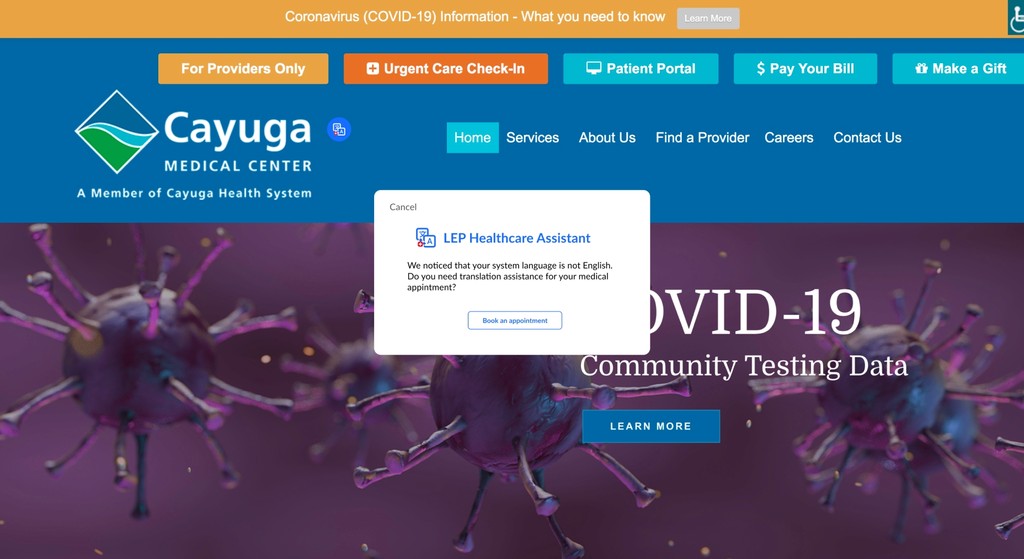
Promote the product offline by posting the product information at some medical service institutions, such as hospitals or local clinics.
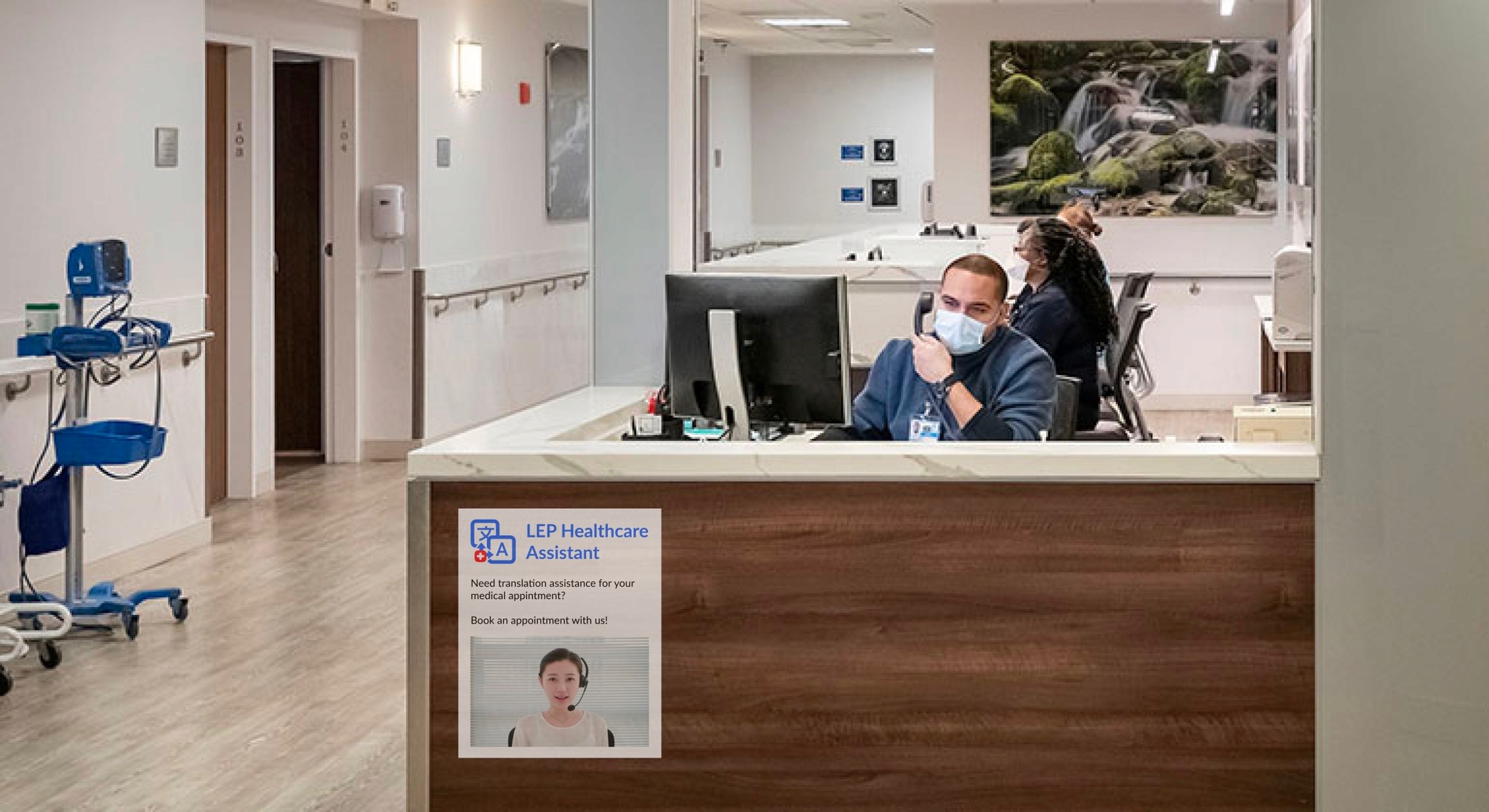
Setting up a product website to further introduce and explain our service content
REFERENCE
Anastasiou, D., & Gupta, R. (2011). Comparison of Crowdsourcing Translation With Machine Translation. Journal of Information Science, 37(6), 637–659. https://doi.org/10.1177/0165551511418760
Blumenthal, D. S. (2007). Barriers to the Provision of Smoking Cessation Services Reported by Clinicians in Underserved Communities. The Journal of the American Board of Family Medicine, 20(3), 272–279. https://doi.org/10.3122/jabfm.2007.03.060115
Dehlendorf, C., Bryant, A. S., Huddleston, H. G., Jacoby, V. L., & Fujimoto, V. Y. (2010). Health Disparities: Definitions and Measurements. American journal of obstetrics and gynecology, 202(3), 212–213. https://doi.org/10.1016/j.ajog.2009.12.003
Driving Innovative Health Care Solutions for Underserved Populations. AAMC. (2019, July 10). Retrieved from https://www.aamc.org/news-insights/driving-innovative-health-care-solutions-underserved-populations.
Wilks, Yorick (1979). Machine Translation and Artificial Intelligence. Translating and the Computer, B.M.Snell (ed.), North-Holland Company 27-43.
Zaidan, Omar F. And Callison-Burch, Chris. Crowdsourcing Translation: Professional Quality from Non-Professionals. Proceedings of the 49th Annual Meeting of the Association for Computational Linguistics, Pages 1220-1229. Portland, Oregon, June 2011.